Trading in the Age of Data
Are You Data Fluent?
Data management is no longer just for quants; everyone on the trading floor needs to understand the role that split-second data and price discovery play if they are to optimize performance and ensure best execution in a post-MiFID II world.
As more message traffic, order types, participants and behaviours pulse through the market than ever before, ‘business as usual' is no longer a viable option. A new approach to leveraging data is needed. For traditional buy-side clients, this can seem daunting. Not only are best execution requirements more rigorous under MiFID II, but the mind-bending pace of technology development has tilted the competitive advantage toward firms with greater commitment to quantitative analytics and IT investment.
While some quant models focus on market trends over hours, days and weeks, others are employed at speeds well beyond the ability for humans to comprehend, seeking to eke out small gains, or “micro-alpha” through pricing anomalies or discrepancies. Data suggests the trading strategies of these electronic market makers and statistical arbitrage firms help to tighten spreads and smooth out temporary imbalances, while others still argue they do little but create a mirage of phantom liquidity. Understanding how these market making strategies play out is an important first step, but buy-side traders in particular, if they are to optimise this liquidity, should do more; they should seek out ways to level the data playing field.
There are many ways to do this, but they all include the need to access high-quality real-time trading data – and the ability to curate, process, analyse and act upon it while avoiding the inherent biases that exist in human behaviour.
Key Takeaways
- Data-driven approaches are needed at all levels of market interaction.
- The proliferation of venue types and messages requires a new approach to complexity.
- Data quality has many aspects – and is a prerequisite for many trading tools.
- Next-gener ation SORs must incorporate low-latency technology with multi-phase decision-making.
- Workflow automation allows objective broker assessment and improves high-level monitoring.
The Data Investment Imperative
Given the huge advances the industry has made in processing speed, data storage, and visualisation tools, the trader who hits the keys today has more power than ever. But that same trader also has more risk than ever. In today's world, it's not good enough to passively assess TCA and opportunity cost at the end of the day or month; we are entering an environment in which “at-trade” optimisation is necessary.
Trading systems must now analyse real-time data from multiple lit order books, from bilateral SI quotes, from dark trades under different waivers, from auctions lasting fractions of a second, and from IOIs with hidden criteria. At the same time, they must incorporate factors such as optimal choice of order strategy, changing market conditions, availability of liquidity, signalling risk, estimated costs, probability of completion, and many other elements. And all of this in sub-second time horizons.
For many firms without dedicated technology teams, it's simply not possible to analyse terabytes of data and millions of price points throughout the trading day from such an array of sources. So they are faced with difficult decisions about how, where and when to invest in the technology arms race. For investment banks, there is little choice but to spend millions on proprietary tools to maintain a competitive offering, but for institutional clients the decision is often less clear-cut.
Regardless of the technology budget, excellent performance requires the speed and quality of the data to be not only timely and accurate, but also highly-tuned to individual needs.
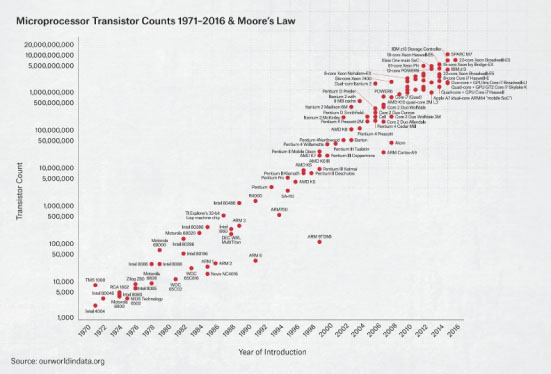
Too Much of a Good Thing?
The term 'Big Data' has been used as an umbrella term for many vague concepts, but in today's fragmented equity markets the sheer scale of information should not be underestimated because it is, indeed, big. Volume, velocity and variety are commonly used as data metrics, but quality and quantity are really what you should be looking out for.
Quality
- Data quality changes over time as new labels and paradigms are applied.
- Storing packets of historical information may create more problems than it solves: how can you be sure that you've got the right level of details for the needs you may have in 12 months' time?
- Differences in the speed of data transmission between locations, and between decisions and actions, have become as magnified in importance as real-time pricing. This means that data are often stale before they even arrive at your location.
- The range of data to be considered is not standardised; price is just one component. Trading styles, strategies, models, and other parameters affect many different aspects of execution. A participant may need to consider many different types of inputs at different times to accomplish different goals.
- The number of factors that must be incorporated into today's models has grown exponentially in recent years, along with the number of ways that automated order types are able to react. In a bilateral, customised world where different streams of prices may be tailored to different objectives, how do you evaluate whether you're looking at the right ones?
Quantity
- The significant technology cost of managing huge volumes of pricing data is a key reason why many financial firms look to partner with independent firms.
- Co-location-related minutiae that may have seemed esoteric five years ago must now be taken seriously as timestamps increase in granularity and microseconds—or even nanoseconds—become the norm.
- How do you know if you are processing in real-time or whether your feeds are sufficiently synchronised? Because that is what it takes to be swift enough to capture liquidity that is fleeting, and to manage your queue priority optimally.
- Data rate refresh periods are now so fast that updates can occur in less time than it takes light to travel a few metres.
- In the space of one millisecond (the time for a typical photo flash strobe), a stock may see its price change up to 100 times or more – what happens if you can't rely on the data?
Building Smarter Smart Order Routers
Absorbing and reacting to the stream of live market data is usually the job of a brokerage firm's Smart Order Router (SOR). No longer a simple venue router, it now has to also process data from customised bilateral liquidity sources and be able to act on the appropriate feed. This requires a fresh set of eyes and the use of more powerful technologies:
Co-location puts your trading system next to the trading venue's matching engine.
GPS time synchronisation ensures all systems speak to each other efficiently and are aware whether their data are up-to-date with a high degree of precision.
Low-latency high-bandwidth fibre optic networks ensure that market data and orders are passed between co-location venues as quickly as possible without the risk of data being “queued” on its way between locations.
Market data normalisation systems such as a “field programmable gate array” (FPGA) rapidly deliver market data to the heart of the SOR engine.
High-capacity order entry platforms lift the load of protocol normalisation from SORs, providing the ability to increase messaging rates beyond those offered as standard by a trading venue.
External latency monitoring systems provide feedback on the health of the system and ensure that it is monitored for harmful increases in latency and drops in processing throughout.
Integrating these tools into a venue selection process requires a layer of ultra-fast logic, combining pre-trade checks and risk controls with a multi-phase, multi-parameter approach. Venues offering price improvement at the expense of speed may need to be ignored; likewise certainty of a fill may be preferred to reducing one's footprint. Some feeds should be mutually exclusive, and others accessed in parallel. The only way to navigate it all is through the data.
Transaction Cost Analysis (TCA) is where the results of any changes to behaviour are monitored and analysed – and where the efficacy of the SOR is understood. Without a robust framework, and high-quality, reliable data, it is impossible to use a scientific methodology and run the statistical analysis required to make meaningful business decisions on changes to algo design or routing policies.
Time synchronisation
Venues and HFTs must operate with a precision of 1 microsecond, accurate to within 100 microseconds and traceable to UTC.
Market data
Disaggregated and non-discriminatory market data feeds are required from venues. Firms registered as SIs need to make public quotes.
Co-location
Venues are required to offer non-discriminatory and equalised access in co-location and proximity hosting including equal length cabling between the user and the venue.
Access to CCPs
Venues must provide access to their trade feeds to allow competitive clearing.
Order recordkeeping
Venues and financial firms must provide accurate records of all their internal system decisions for matching engines and algorithms.
Best execution data
Venues and financial firms must provide quarterly best execution analysis.
Creating a New Generation of Intelligent Automation
Digitisation is ever-present in our daily lives, and is particularly prevalent on the trading floor. Along with recent advancements in processing power and order management technology, the focus now is on using automation to help traders identify alpha, reduce errors and adapt trading strategies in an opportunistic way. One type of workflow automation process is known as the broker or algo “wheel”.
Early iterations of this kind of technology failed to catch on very broadly, perhaps because they were not as transparent as most buyside clients would have liked, and they interfered with the buyside/broker relationship. These early wheels took over the clients' discretion and instructed the brokers how to modify their algos and venue routing patterns in order to suit their own logic. But newer variations on automated, randomised technology like this could become more popular. These new broker wheel solutions provide automated broker selections according to configurations set by the client without imposing requirements or disintermediating the executing broker. The wheel does not replace humans; it makes it possible for them to compete better through automation, but unless it can demonstrate that it consistently makes the right choices, its use is limited.
A broker wheel needs to be more powerful than simply a routing machine, however. It should provide the tools to measure broker performance, simplify tickets and facilitate broker-to-broker comparisons, using tools like randomisation and A/B investigations. Most clients are not looking for an ever-increasing number of algos, but they are looking for consistent execution and the ability to monitor and measure execution quality.

The Importance of Continual Forensic Analysis
Systematic solutions speed up information processing and increase order handling efficiency, but are not completely without drawbacks. The lack of direct human involvement can allow unchecked mistakes to be replicated an infinite number of times as machines follow their pre-programmed behaviour, and even tiny configuration changes can have a significant impact on performance outcomes over a long period.
As the market landscape changes, systems need their assumptions continually challenged if they are to remain relevant. A key element of monitoring the reaction of systems is the maintenance of accurate independent models of expected behaviour, which allows for continuous benchmarking against both historical trends and expected theoretical outcomes.
The requirements for post-trade TCA data are also evolving. Standardised reports that highlight the same trends quarter after quarter must be replaced with new interactive tools that allow users to ask questions - if the numbers have changed, why have they changed? If not, why not? Finding new angles on old datasets is a vital part of the TCA process.
A good platform should allow the creation of an ad-hoc set of analysis metrics, which should be accompanied by a similarly flexible set of visualisation tools. It may involve creating a top-down view of all trades subdivided by custom classifications, or a bottom-up approach with a set of filter thresholds, or a time-series, or combination of all these. Most importantly, the practice of generating new ideas should be built into the process framework wherever possible, and regular reviews setup to ensure continued relevance and improvement.

Changing Your Relationship with Data
Data empowers astute decision-making on the trading floor, and will separate leaders from laggards. Without access to high-velocity, clean data fuelling best execution and performance-driven decisions, investment firms are going to struggle to compete for hard-to-find liquidity and deliver on their regulatory and investment objectives. The way to ensure consistent execution quality is to remove human bias where it interferes with efficiency, employ data-driven models that learn as they go, and accelerate your decision-making capabilities. The role of the next-generation trader will increasingly be to examine and authenticate his or her own automated tools by continually processing TCA, asking the right questions of their data, assessing market conditions, challenging models and assumptions, and evaluating the ways the systems respond to them.
For investment managers, it is often a difficult decision: should I build out my desk quants and my internal technology stack, or is it better to partner with a third-party that is already set up to deliver these capabilities? The trade-offs will depend on the size and nature of the firm, and on its underlying objectives. But going forward, all traders need to ensure that the decisions they, and their systems, make are based on strong, reliable, high-quality data.
Turning data into intelligence – how it is analysed and used – is front and centre to achieving best execution, both in the regulatory sense of taking “all sufficient steps” and in the real sense of optimising bottom-line investment performance. Becoming data savvy is no longer the purview of the quant analyst; it's everyone's responsibility.

Benjamin Stephens
benjamin.stephens@instinet.co.uk
T: +44 207 154 8743 Salvador Rodriguez
salvador.rodriguez@instinet.co.uk
T: +44 207 154 7252